In a world abuzz with AI capabilities it is sometimes difficult to know how to get started. Vendors...
Unlocking CRM Analytics: Practical Strategies for a Messy, Multi-Platform World
I've struggled over the years with setting the right strategy for CRM Analytics. There never seemed to be a neat answer. In this piece I discuss some of the options and offer guidance for what to do.
We all want analytics, don't we? We want to unlock the value in our enterprise data to drive better efficiency and effectiveness. We want to respond to changes and feel confident that our decisions were based on hard data. We also want analytics for everyday operational needs too, such as understanding what our cash flow looks like, or if we are going to run out of stock based on expected orders. Increasingly we want to manage to guard bands and so we want to know when we are going out of bounds.
So ideally we have all the transactional data in one place and we run our analytics and AI there too. The trouble is that no organization has one platform for everything and the datasets are not in one place. Worse, they are all structured differently so it is not trivial to connect them. Even in my small consultancy I have three major platform providers. I can connect the data as I have been careful to establish which is the authoritative source, but the idea of running all my analytics in one singular spot is elusive. It simply depends on what analytics I need.
So in this area the best answer is "it depends" and your to-be architecture is typically going to be a bit messier than we would like.
Set the strategy on your CRM analytics hub by creating clarity on the key analytical use cases you need to satisfy, but lay an anchor to windward…
So what are some common approaches?
Use your CRM
Not just your actual CRM, typically, but the analytics hub that has been brought/grown into it.
These days they can smartly take data feeds from outside of the CRM and offer advanced analytics, boosted by the AI implementations of your CRM vendor. Your CRM vendor is investing heavily in this area.
That gives you good integration with the CRM workflow and therefore positions you well to deploy the actionable insights across your organization, especially as you have already integrated all material customer-related transactions into your CRM, or are on the way to doing that. The line between in-app transactions and analytics is blurred, satisfyingly. AI is embedded and helps to join the dots so your team can see insights right there when they are looking at the related records. An example of this would be an AI-assessed Opportunity Score that is embedded into a Pipeline View that is embedded into the Sales CRM.
On the downside it means you are locked into the flexibility (or lack thereof) of your CRM's analytics offering and, additionally, the fees may be high, especially when you take into account the extra CRM seats you now need to allow the rest of the organization to access the analytics in real-time. I did mention that your CRM vendor is investing heavily in this area? They want their ROI.
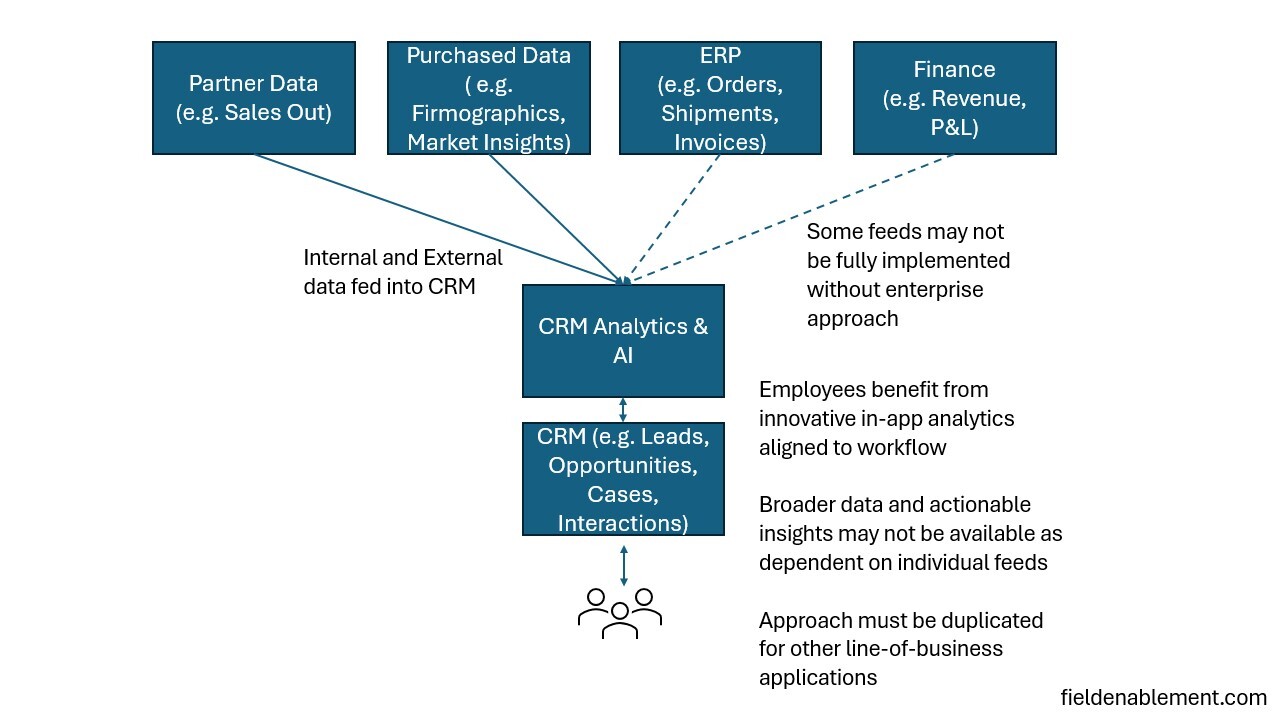
[Figure 1 - Use your CRM and its analytics offering]
Use your ERP or other major platform established in your organization
As above.
Use a separate analytics hub
This is the traditional approach. Recognize that no single enterprise application has all the data you need for broad analytics. Pipe all your data to one hub, which will be a best-of-breed analytics solution, so will have amazing facilities for importing and organizing the data and processing it with an array of analytical tools, including continuously-improving AI.
You have to spend money and effort on creating the hub. How this looks compared to the CRM/ERP data hub approach depends on the stack you have in your enterprise. It is worth making the comparison as part of setting your strategy.
There is nothing you cannot do with these tools. And for breadth, you are now looking across the entire enterprise dataset and can gain broad insights from that position.
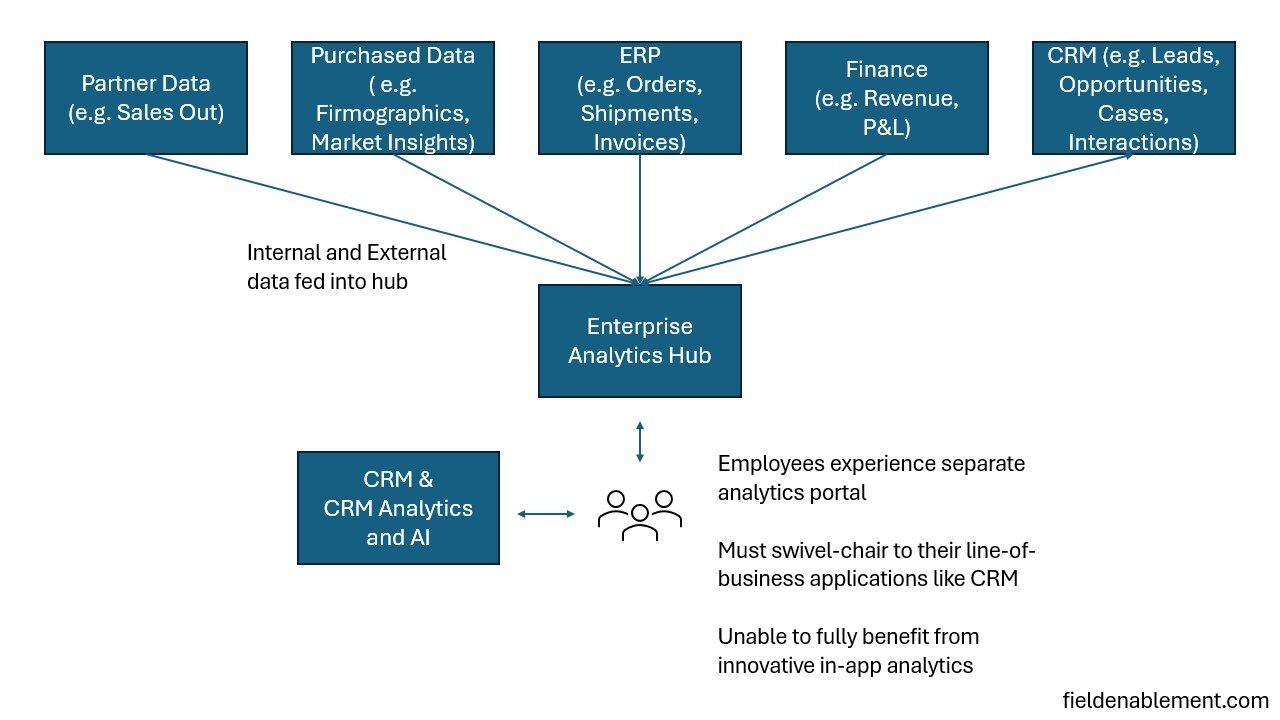
[Figure 2 - Centralized Enterprise Analytics Hub]
But.. the purpose of analytics is to drive actionable insights such as:
- How much of each product must the Factory build over the next six months?
- Which customers should receive the most focus from the Sales Team?
- Which customer segments should receive which messaging from Marketing?
- Which product lines and customer segments are growing, which are shrinking and therefore how must we position our overall business to stay relevant and capture these trends?
Most of these insights then have an impact on a workflow that is conducted within one of the other enterprise applications… so the actionable insight you generate from your analytics must somehow come back to those applications. In the examples above that might be:
- Build plans, buy plans
- Sales coverage lists, territories, quotas, account teams, commission payments
- Market segments and campaigns
- New product introductions and retirements. New product development. Workforce shifts.
Too often, then, there is a gap between the analytics that pour out of the hub and the actual actions that happen in the organization. At best this can look like swivel-chair inefficiency and at worst it means that the analytics were pretty pictures that drove no action. This is in sharp contrast to the embedded insights in the in-app approach.
Shame about all the extra money and effort spent on that hub, eh?
Data Mesh
An approach that recognizes that one huge enterprise data hub involves a level of co-ordination that humans find challenging. Instead, implement a number of data hubs that are managed by the owning organisations to agreed quality standards. Think Sales, Marketing, Finance, Manufacturing. All contribute to the enterprise. This gives agility and focus at the organization level whilst still providing an enterprise overview. In some ways this is what tended to happen with practical enterprise data hub implementations.
The challenge is ensuring all organizations keep pace and implement to sufficient standards to be able to meaningfully contribute to the enterprise level. This requires a level of enterprise agreement and co-ordination that was challenging in the first place when creating a centralized hub.
For me this is a practical approach to implementing a hub for very large organizations, but we still need to get the insights back to those who can use them.
Hybrid
It took me a long time to embrace this. There isn't really an alternative, because no organization has all its transactions in one platform and you want to deliver the insights where the people are. For me it is the practical approach.
- Keep relevant analytics embedded in the enterprise applications themselves, e.g. in the CRM, ERP, dedicated exec dashboard
- Make sure the enterprise applications have the data they need for their analytics to work properly. For example your CRM needs revenue data which might come from the ERP. Your ERP needs Quote data that might come from the CRM. Accept the uncomfortable truth that there will be overlap in use cases and data as all vendors broaden their offerings, especially with AI.
- Also have an analytics hub for complicated, broad analytics. It is expensive, but if you don't have a hub it will take you too long to compile the data when you really need it. Unexpected things will happen in the world and you will want to be able to react quickly to make data-informed decisions. Covid was a good example.
- Use the hub and governed APIs to pipe data between the applications when they need it. This will help keep to one version of the truth. You can also abstract the data which can help with security and privacy.
- Try to keep the moving parts down to the minimum.
- Be clear as to which system is the authority on which topic to avoid inefficiencies through friction in the organization.
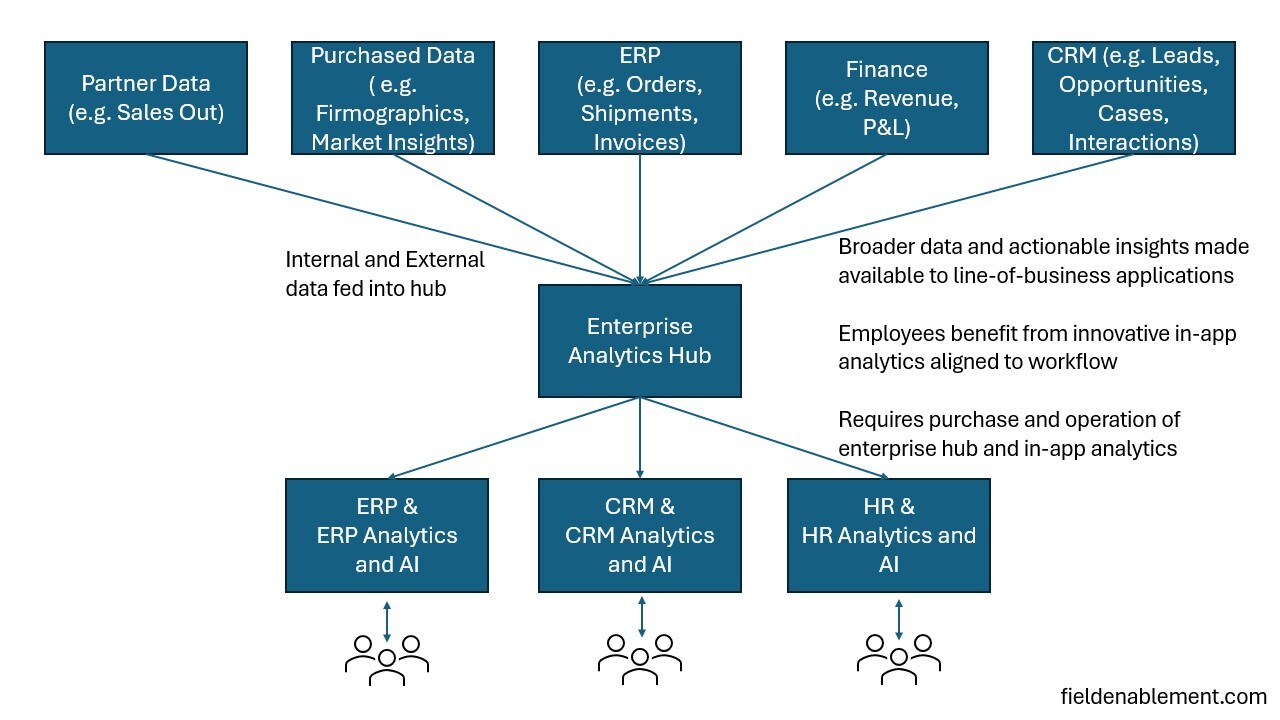
[Figure 3 - One example of a Hybrid approach to enterprise analytics]
What to do?
As ever, creating as much clarity about your key value drivers at an enterprise level will help to guide the decision here. I think many enterprises will land on a Hybrid approach (Figure 3) as the most practical, although if you do have one platform that dominates in your organization you might be able to use that (Figure 1).
I've talked about Benefit Maps before and they can apply here if you think at a whole-enterprise level. Essentially, develop a clear understanding of the business benefits you are looking to drive and, here in particular, the way you will measure them. Those ways of measuring define some of the analytics you need. For example, you might have a strategy to acquire new customers… so now you need to work out what counts as a new customer and how you are doing across the enterprise.
Without such an approach you risk trying to serve every potential possible use case and go around in circles.
Invest in Data Quality. This doesn't mean clean it up once and then it is tidy and actionable forever. You have to invest in ongoing data-governance standards and processes to keep data compliant with these standards. AI will help the people doing this, but it won't reliably do it for them - at least not yet. Try not to see this work as a tax, rather a strategic enabler for your enterprise agility and decision-making.
CRM and Enterprise Analytics are tricky areas, especially as these are strategic investments that are difficult to change quickly. Also, your ability to predict what you will need for the future is lower in this area, given the incredible progress being made with AI. Accept that you need some extra cost and redundancy to maintain enterprise agility.
You can't afford to not be able to deploy AI on your enterprise data for classic analytics, natural-language self-service, visualization and real-time reaction in this highly-dynamic business landscape.
To discuss CRM analytics further please reach out on LinkedIn or on fieldenablement.com
Gareth.